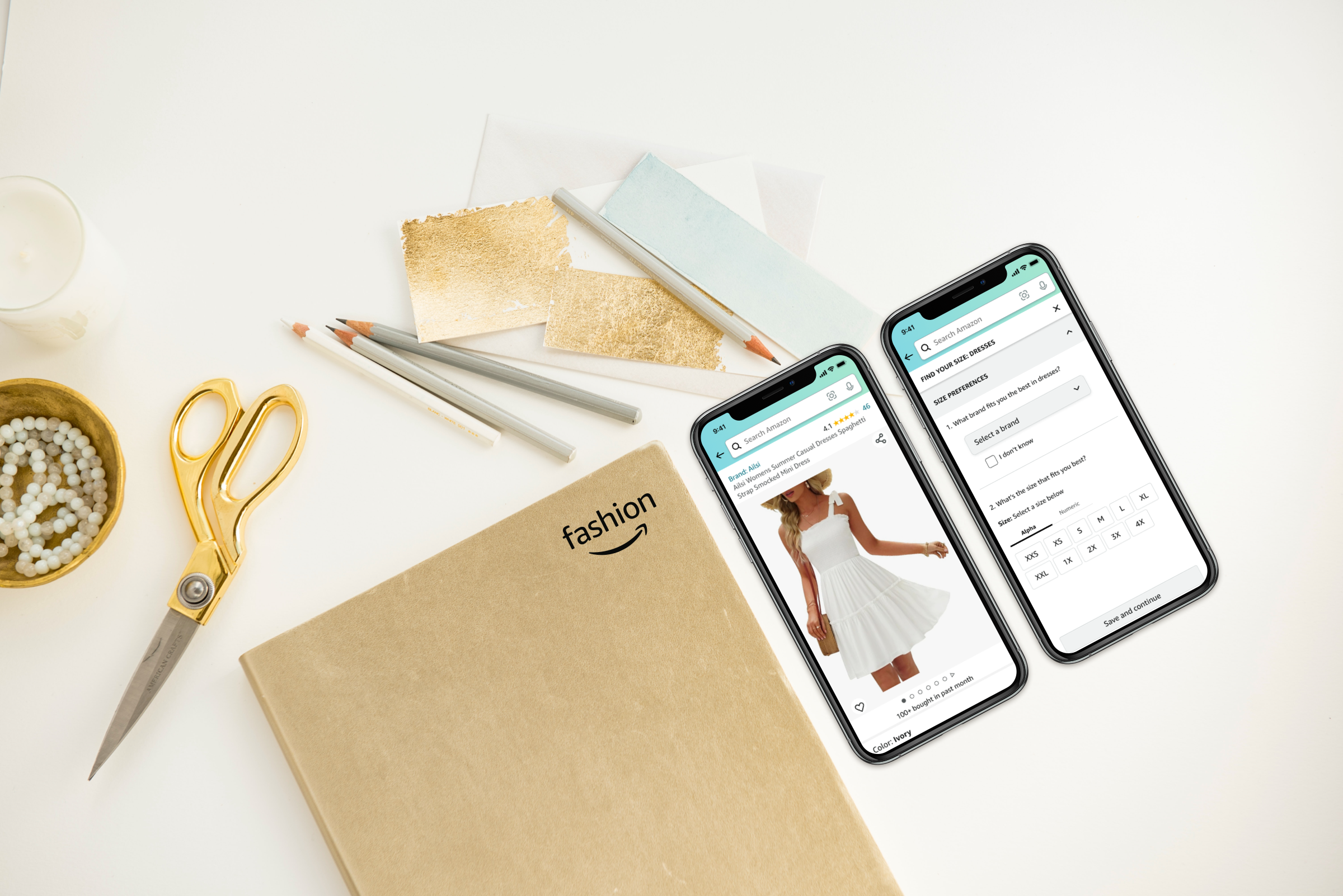
Size FINDER | amazon fashion
UX / UI Design
THE PROJECT
Online shopping for clothes is a $208 billion market in the United States alone. But the reality is that finding the ‘right size’ for every customer remains a challenge across the global fashion industry. No ‘one size fits all’, as every body is different. Poor sizing of an item leads to dissatisfied customers, customer returns, and most importantly: decreased trust in an apparel brand or retailer.
Amazon’s goal was to increase size recommendation coverage from 25% to 90% of the top 20 product categories.
Thus, a fit data collection experience like Size Finder was required.
TEAM & ROLE
Everyone involved in this project was from Amazon Fashion’s (Softlines) Fit team. Fit is a core team dedicated to Amazon’s size charts, guides, and customer sizing across several apparel and accessory categories.
I worked with Product and Engineering teams on a daily basis, with my Design manager overseeing the work. My primary responsibilities:
I designed initial mocks, iterations, and hand-off specs for internal web lab testing.
I conducted extensive competitive audits (44 brands) and helped with collecting user research on the initial CX flow.
When other designers took over closer to the product launch; I played more of a supervising role.
Other teams involved: Fit REX, Vikings, Science, Quality Assurance, Business Intelligence, Profile, and PSPW
PLANNING
Size Finder was a product of Amazon’s customer obsession and earn trust leadership principles, among other team tenents. Our competitors included companies such as TrueFit or Fit analytics that had already pioneered ‘finding the right size’ for apparel retailers.
I met with the product team to learn more about the project’s history.
I looked at 44 brands during a competitive audit, collecting data on the fashion industry standards for size charts, reviews, and size recommendations.
Size recommendations could not be offered to new customers, or Amazon customers with insufficient purchase history.
Recommendations were based on machine learning models that relied on data from previous apparel purchases. e.g. a customer who had bought a dress in Large, was likely to be a Large in other brands.
BENCHmarking
As Size Finder had yet to launch, there was limited user research. Any information that could boost customer impact and engagement metrics was valuable. I conducted competitive audits on fit features from 44 competitors. These audits included:
Size Finder clothes competitive analysis (Mobile and Desktop)
Size Finder shoes competitive analysis (Mobile)
Shein Dive Deep (Mobile)
Body shape visuals across the fashion industry (Mobile)
We analyzed established fashion brands such as Uniqlo, Gucci, Madewell, as well as independent retailers such as Revolve, Savage Fenty and Pepper.
research
The Fit team tried different survey styles, size blurbs and other UX design explorations before creating Size Finder. When I joined the team, they were analyzing user research and consumer behavior, which included key points:
Many customers shopped according to brands they recognized, not size
Many customers did not trust size charts to be accurate
Most customers did not know their measurements or have access to measuring tape
Most customers trusted customer reviews over size charts
Lack of diversity and inclusion was also mentioned in several quotes
Specific stats unavailable; Amazon confidential information
Size Finder was targeted at new customers unfamiliar with Amazon brands. Therefore, we brainstormed a flow that began with a customer’s preferred brand, and converted it to an equivalent size of the Amazon brand they were considering. This would help us Earn Trust, and stay true to Customer Obsession leadership principles (Amazon LPs).
How this was done via machine learning:
“Once a brand mapping was established, we used an [machine learning] model trained on our catalog purchase data to identify the best-fitting size in the target ASIN based on ASIN attributes such as style, fabric, How it Fits, and, Marca score. This two-step approach enables us to provide a more accurate size recommendation when customers choose to provide their best-fitting brand.”
Some questions we went over during our initial designs:
What if someone doesn’t have a favorite brand?
Is a customer’s weight necessary?
Would a weight slider or keyboard input be more intuitive?
Which writing tone was better? e.g. ‘customers like you’ vs. ‘customers with your size’
We also chose to launch Size Finder with Women’s Dresses, the product category that had the highest number of returns within the Amazon Fashion department.
APP USERS
“I am unsure [about fit] because stores like Amazon that have like a million different sellers — I never know if the fit is going to be right. I know my sizing at a store like Torrid. I know it because it's a specific store for my size that I shop at. Amazon is always sort of 50/50 whether it's gonna fit.”
- Amazon Fashion customer who shops in the plus size department
DESIGN Process
My design manager and product team had curated a list of requirements, objectives, and initial flow, but the screens were not yet complete. My process included the following:
Reviewed existing documents, designs, and user research
Conducted 3 competitive audits on 44 fashion brands, benchmarking our Fit features to learn what we did well, and what we could improve on
Designed initial mock screens and rounds of iteration after feedback
Implemented new UI e.g. the Size Finder ingress icon, global measurement unit toggle buttons
Prototyped different flows for user testing and research (Invision, usertesting.com)
Compiled our user research with key insights and next steps for design and product
Organized design assets, flow, and specifications for hand-off to engineering
Reviewed with other designers and drafted mocks for a Size Finder desktop version
Size Finder would go through rounds of internal beta testing (web labs, dial ups), leadership reviews and QA checks before launching across apparel product categories in USA and Canada.
SITEMAP
The design team determined the flows:
SKELETON UI
WIREFRAMES
USER EXPERIENCE
INTERFACE DESIGN
Following the Softlines design library (Amazon Fashion), I kept assets to mostly black and white colors and the Amazon Ember font family.
Size Finder’s ingress was restricted to around 100px in height, with a fixed location between the Color Twister and Pricing. Fit tested different ingress icons, such as using a measuring tape or ruler to indicate ‘find my size.’ However, they received mixed results as users associated those icons with a size chart or virtual fitting room. We eventually chose a sparkle icon from Noun Project, with some modifications for our use case.
A few components such as the weight slider had to be designed and built from scratch. Some apparel sizing such as men’s pants, came in a variety of sizes or formats (numeric/alpha). In order to keep the screen height short and information easily digestable, I organized the size buttons into tabs. Selecting one size would automatically convert to the corresponding size on the secondary tab.
Additional UI component work:
Measuring guide UX as a visual aid ‘how to measure’
Updated global size charts
Added a new cm/inch toggle
Added custom icon, referenced Rio design system (Amazon Shopping, Amazon Fashion)
THE CHALLENGES
Keeping the Size Finder survey as short and accurate as possible.
Research showed that users often abandoned the journey after the third screen, so the data collection had to be very minimal, even though more information sharing would lead to better results.
Increasing brand trust with third-party sellers.
Many customers were wary of foreign sellers and did not trust size charts, especially those in the ‘plus size’ product category.The person using Size Finder was not always the end recipient.
Parents tend to buy clothes for their kids on their own account, affecting recommendation results.Customers wanted to know the logic behind the recommendation.
Displaying their regular size led to customers doubting the purpose of the recommendation. Some customers did not want to see the recommendation at all.
FINAL DESIGNS
THE PLATFORM
Designed for Amazon’s mobile app as well as mobile browser, the survey depended on machine learning to provide the most accurate results. The Fit Science team invented a machine learning approach that used external size charts of customer’s preferred brands, and compared them to high-quality size charts of brands in our catalog (e.g. Amazon Essentials). Machine learning also took into account attributes such as style, fabric, and customer feedback. This enabled customers to receive size recommendations while they browsed other items in the same department - and in relevant Amazon Fashion experiences such as Profile hub.
RESULTS
Size recommendations were forecasted to contribute to $557M in attributed annual revenue (based on weblab data) by alleviating fit anxiety and boosting customer confidence in making a purchase.
Excerpt from weblab data:
Since collecting optional height and weight on detail page and customers reviews, 33M customers (which shows up on 60% of apparel reviews) have shared their height and weight information, which is now stored in their profile for future size recommendations. At the same time, innovative retailers across the industry have also launched compelling experiences to collect fit information (e.g., GOAT, Rent The Runway, Zara, Fit Finder-acquired by Snap) and across the industry, we see that customers are willing to provide personal information to power personalized shopping experiences.
From the Fit Benchmarking study I did, we saw that 35% of our competitors have already launched a more comprehensive and compelling customer data collection CX than our previously launched height and weight cards. Size Finder will be a critical feature for customers worldwide to find the right size, quickly and accurately.
Set to launch in 2023, with the North America region first, Size Finder would eventually be rolled out to Amazon’s worldwide marketplaces. We expect the $557M to grow with the online shopping industry, especially with research and improvements at every phase.